728x90
● 2단계 코드 복습
import pandas as pd
iowa_file_path = '../input/home-data-for-ml-course/train.csv'
home_data = pd.read_csv(iowa_file_path)
● 판매 가격에 해당하는 변수를 y라는 새로운 변수에 저장하기
→ SalePrice는 우리가 예측하고자 하는 값으로서 출력 데이터에 해당됨
y = home_data.SalePrice
● 모델이 학습하기 위해 사용하는 입력데이터 입력
→ 'LotArea', 'YearBuilt', '1stFlrSF', '2ndFlrSF', 'FullBath', 'BedroomAbvGr', 'TotRmsAbvGrd' 와 같은 주택 특징들이 있음
→ 읽어온 데이터에서 우리가 예측에 사용하고자 하는 주택 특징들만 선택하여 새로운 DataFrame인 X를 만드는 작업 수행
feature_names = ['LotArea', 'YearBuilt', '1stFlrSF', '2ndFlrSF', 'FullBath', 'BedroomAbvGr', 'TotRmsAbvGrd']
X = home_data[feature_names]
→ X를 출력하면 다음과 같이 나옴
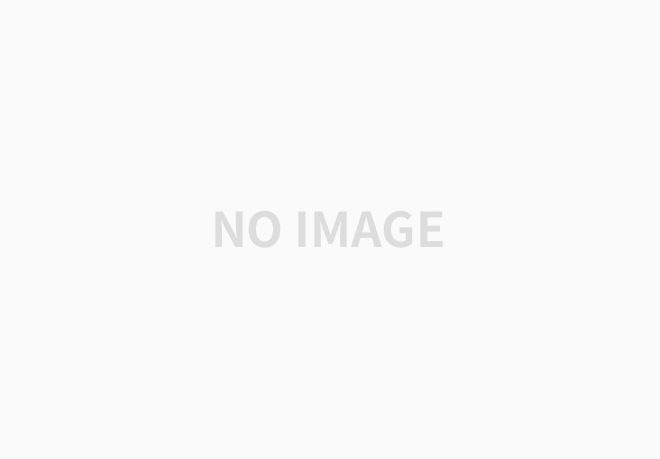
● Decision Tree Regressor 모델을 활용하여 주택 가격을 예측하는 iowa_model 학습
→ DecisionTreeRegressor 모델을 불러와 iowa_model이라는 변수에 DecisionTreeRegressor 모델을 생성
→ 모델은 학습 데이터를 기반으로 학습하고, 주택의 특징들과 가격 사이의 관계를 파악함
→ 학습된 iowa_model은 새로운 입력 데이터를 주입하여 주택 가격을 예측하는데 사용할 수 있음
from sklearn.tree import DecisionTreeRegressor
iowa_model = DecisionTreeRegressor(random_state=1)
iowa_model.fit(X, y)
● 'X'에 대한 예측 수행하기
predictions = iowa_model.predict(X)
print(predictions)
728x90
'Kaggle > Intro to Machine Learning' 카테고리의 다른 글
6. Random Forests (0) | 2023.08.11 |
---|---|
5. Underfitting and Overfitting (0) | 2023.08.04 |
4. Model Validation (0) | 2023.08.03 |
2. Basic Data Exploration (0) | 2023.08.02 |